This domain (www.cognifyhub.net) is for sale. for inquiries, please email inquiry@ginoborlado.org
How AI Is Transforming Disaster Response in Urban Areas
Discover how AI is revolutionizing disaster response in cities, from predicting hurricanes to coordinating relief efforts. As climate change intensifies, AI-driven systems are enabling faster, more accurate responses to natural disasters worldwide, bridging data gaps and enhancing forecasting precision. This new approach, supported by both global organizations and private sector partnerships, offers a powerful toolkit for urban resilience.
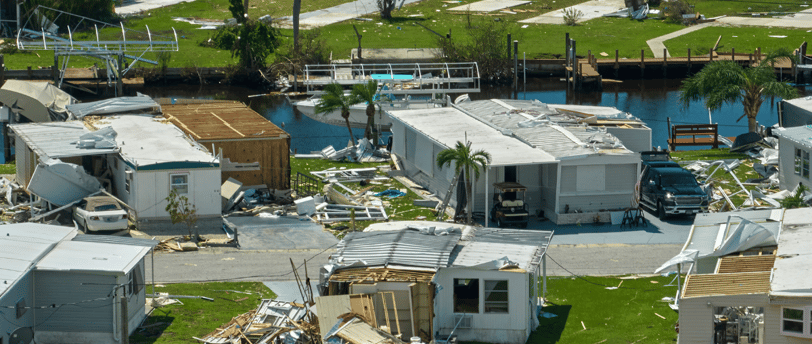
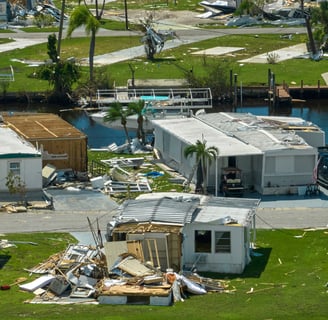
How AI Is Transforming Disaster Response in Urban Areas
With urban populations expanding rapidly over the past 50 years, cities face greater risks from natural disasters. As climate change continues, extreme weather events are becoming stronger and more frequent, increasing the urgency for improved disaster monitoring and response systems. To meet this need, researchers and organizations worldwide are advancing the use of artificial intelligence (AI) in disaster response, aiming for faster, more cost-effective, and accurate approaches.
This week, the Barcelona Supercomputing Center in Spain will host the first meeting of the Global Initiative on Resilience to Natural Hazards through AI Solutions. Launched by the United Nations, this initiative seeks to guide governments, organizations, and communities in leveraging AI for disaster preparedness and response.
Building a Framework for AI-Enhanced Disaster Management
The groundwork for this initiative began almost four years ago, with contributions from the International Telecommunications Union, the World Meteorological Organization (WMO), and the U.N. Environment Programme. Their early efforts focused on defining best practices for integrating AI in disaster management, including improved data gathering, forecasting accuracy, and communication channels.
AI’s flexibility offers a range of applications for disaster preparedness. For instance, AI models helped map areas prone to road blockages after hurricanes in Tallahassee, Florida. In another case, AI-based weather forecasting accurately predicted Hurricane Milton’s landfall in Florida, aiding in targeted preparations. In collaboration with AI translation services, the National Weather Service has even begun offering hurricane warnings in Spanish and simplified Chinese, reducing translation time from an hour to just 10 minutes.
Coordinating Response with AI Analysis
AI is also instrumental in coordinating disaster response. Following hurricanes Milton and Ian, the non-profit GiveDirectly employed Google’s machine-learning tools to assess satellite images before and after the storms. This enabled quick identification of the worst-hit areas and efficient prioritization of financial aid. Similar AI-based image analysis was used in Quelimane, Mozambique, after Cyclone Freddy and in Adıyaman, Turkey, following a severe earthquake.
Governments usually operate early warning systems, but private companies are increasingly involved in AI climate modeling. Start-ups like SeismicAI are working with local agencies in Mexico to deploy real-time AI-powered earthquake detection networks. Major tech companies, including Google, Nvidia, and Huawei, are collaborating with European forecasters to produce accurate medium-term weather forecasts, which are faster and less resource-intensive than traditional models. IBM and NASA recently released an open-source model for diverse climate applications that can run on standard desktops.
Improved Forecasting with AI Technology
AI-enhanced forecasting models have been part of weather prediction for years, but recent developments are reshaping the field. Traditional models use complex physics equations to simulate the interactions of air and water in the atmosphere, which can take hours to process even with supercomputers. In contrast, AI models analyze patterns from decades of climate data, mainly collected via satellites and ground sensors, to generate faster, more detailed forecasts.
AI-based models create a finer-grained resolution than physics-based models, which helps allocate resources more effectively during extreme weather events, particularly in cities. Johan Mathe, co-founder of the forecasting startup Atmo, noted that their models, used in the Philippines and Tuvalu, break down geographic areas into grids as small as a square mile, enabling precise responses in affected urban areas.
Challenges and Ethical Considerations
The effectiveness of AI models depends on the quality of the data they are trained on, which can be inconsistent in lower-income areas that often lack reliable weather sensors. AI models trained on incomplete data may be less accurate in predicting extreme weather where it's most needed. Additionally, AI models can function as “black boxes,” producing results without fully transparent reasoning, which raises concerns about accountability and reliability in high-stakes situations.
To address these issues, the U.N. initiative will test its guidelines in real-life settings. The Mediterranean and pan-European forecast and Early Warning System Against natural hazards (MedEWSa) is set to apply AI for wildfire and flood prediction in Greece and Georgia, respectively. These pilot projects will provide valuable insights for refining AI practices in disaster response.
Filling Data Gaps with Private Sector Involvement
Some private companies are stepping in to address data shortages. Weather technology startup Tomorrow.io, for example, has launched satellites equipped with radar to gather meteorological data in regions lacking ground-based sensors. This data supplements their models, which cities like Boston use to determine pre-snowfall road salting schedules.
The Systematic Observations Financing Facility (SOFF), another U.N.-backed program, aims to support data collection in underserved regions. Johan Stander from the WMO, a partner in SOFF, emphasizes the importance of using AI as a support tool rather than a replacement for traditional meteorological services. According to Stander, private companies should bolster public services without overshadowing them, ensuring that human experts remain accountable.